

NOTE: The `word_embeddings` should be generated through `.extract_embeddings` as the order of these embeddings depend on the vectorizer that was used to generate its vocabulary. word_embeddings: The embeddings of each potential keyword/keyphrase across across the vocabulary of the set of input documents. doc_embeddings: The embeddings of each document. seed_keywords: Seed keywords that may guide the extraction of keywords by steering the similarities towards the seeded keywords. NOTE: This does not work if multiple documents are passed.
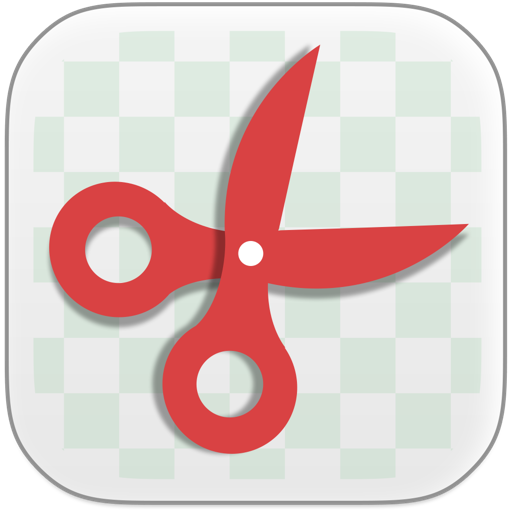
vectorizer: Pass in your own `CountVectorizer` from `sklearn.feature_` highlight: Whether to print the document and highlight its keywords/keyphrases.

nr_candidates: The number of candidates to consider if `use_maxsum` is set to True. diversity: The diversity of the results between 0 and 1 if `use_mmr` is set to True. use_mmr: Whether to use Maximal Marginal Relevance (MMR) for the selection of keywords/keyphrases. use_maxsum: Whether to use Max Sum Distance for the selection of keywords/keyphrases. NOTE: This is not used if you passed a `vectorizer`. top_n: Return the top n keywords/keyphrases min_df: Minimum document frequency of a word across all documents if keywords for multiple documents need to be extracted. stop_words: Stopwords to remove from the document. keyphrase_ngram_range: Length, in words, of the extracted keywords/keyphrases. Arguments: docs: The document(s) for which to extract keywords/keyphrases candidates: Candidate keywords/keyphrases to use instead of extracting them from the document(s) NOTE: This is not used if you passed a `vectorizer`. array = None, ) -> Union ], List ]]]: """Extract keywords and/or keyphrases To get the biggest speed-up, make sure to pass multiple documents at once instead of iterating over a single document. model = select_backend ( model ) def extract_keywords ( self, docs : Union ], candidates : List = None, keyphrase_ngram_range : Tuple = ( 1, 1 ), stop_words : Union ] = "english", top_n : int = 5, min_df : int = 1, use_maxsum : bool = False, use_mmr : bool = False, diversity : float = 0.5, nr_candidates : int = 20, vectorizer : CountVectorizer = None, highlight : bool = False, seed_keywords : List = None, doc_embeddings : np. The following backends are currently supported: * SentenceTransformers * 🤗 Transformers * Flair * Spacy * Gensim * USE (TF-Hub) You can also pass in a string that points to one of the following sentence-transformers models: * """ self. 8 """ def _init_ ( self, model = "all-MiniLM-L6-v2" ): """KeyBERT initialization Arguments: model: Use a custom embedding model. The most similar words could then be identified as the words that best describe the entire document.

Finally, we use cosine similarity to find the words/phrases that are the most similar to the document. Then, word embeddings are extracted for N-gram words/phrases. First, document embeddings are extracted with BERT to get a document-level representation. Class KeyBERT : """ A minimal method for keyword extraction with BERT The keyword extraction is done by finding the sub-phrases in a document that are the most similar to the document itself.
